Smart Algorithms for Safe Distancing in Public Transport
RMV Introduces Occupancy Forecast
How can digital technologies protect risk groups in public transport better while enabling mobility? Based on big data technologies and self-learning algorithms, HaCon and Siemens Mobility have joined together to develop software that predicts passenger numbers. The German transport association Rhein-Main-Verkehrsverbund (RMV) has now implemented the system.
From now on, RMV passengers planning their trip via the mobile website (m.rmv.de) can check the estimated number of passengers along their route prior to departing. Trip plans showing occupancy predictions as “too high” will suggest alternative connections that have a lower estimated passenger count. RMV’s technological partner HaCon has developed the corresponding software together with Siemens Mobility.
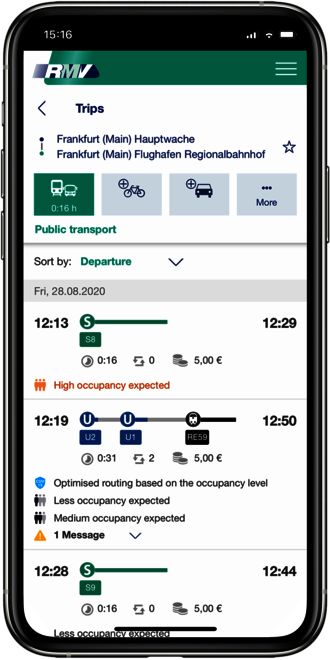
The main goal of the project has been to restore passenger confidence in local public transport. "Our top priority is that our passengers get on buses and trains with a feeling of security – even in times of Corona," said RMV Managing Director Prof. Knut Ringat at the official introduction of the new feature in Frankfurt. "With the new occupancy forecast, our passengers now benefit from a tool that helps them plan their trips even better. Unlike in long-distance transport, we don’t have access to reservation data – this makes us pioneers in Germany when it comes to load forecasts in local public transport."
The occupancy forecast is based on the combination of connection requests from the RMV’s trip planner as well as mobile ticket purchases. The data is stripped of any personal information before it is aggregated and processed; this ensures that the data remains anonymous. In order to make these estimates more accurate, an additional calibration factor is created by using real counting data from the Frankfurt underground trains, trams, buses and the RheinMain S-Bahn. In addition, the self-learning algorithm takes into account predictable external influences such as construction sites and any major events in its forecast. As time goes on and the amount of data increases, the predictions of passenger numbers will become more and more precise.
“The occupancy forecast is a great example of how public transport reacts quickly and effectively to the new challenges posed by the Corona crisis,” says Steven Ahlig, Head of Business Development at HaCon. “With our solution, we ensure that public transport passengers are informed as transparently as possible about how crowded their connection will most likely be, in order for them to avoid large numbers of passengers in the same vehicle. With our optimized routing, we automatically support them in using connections with lower estimated passenger counts."
Screen: Optimized for mobile access, m.rmv.de displays the predicted vehicle occupancy as a pictogram in the connection information. The second option shows an alternative routing suggestion based on a lower estimated occupancy.